The transition of safety responsibility from human drivers to autonomous vehicles (AV) demands not only trust that the system is safe enough at deployment, but also trust that the system will continuously stay safe in a changing traffic environment. This article outlines the main elements needed to enable dynamic assurances for building trust in AV after deployment.
What is dynamic assurance and why is it necessary?
Safety is an indispensable property of autonomous driving, so a systematic approach to safety assurance is mandatory for the adoption and success of AV systems. A key activity in such a systematic approach has to be the explicit design and specification of a comprehensible safety argument backed up by evidence on why the residual risk associated with the deployment of an AV system is acceptable at deployment and will remain acceptable for the lifetime of the vehicle. Since both foreseeable (e.g., changed traffic rules in construction zones) and unforeseeable traffic environment changes (e.g., new traffic actors like e-scooters or changed occurrence frequencies of particular traffic situations) will occur after deployment, mechanisms need to be in place that can collect data to check the validity of assumptions made during development and react if changes potentially increase risk. This means that the AV architecture needs to realize functionality for safety monitoring in addition to the functionality required to bringing passengers from A to B (see Figure 1).
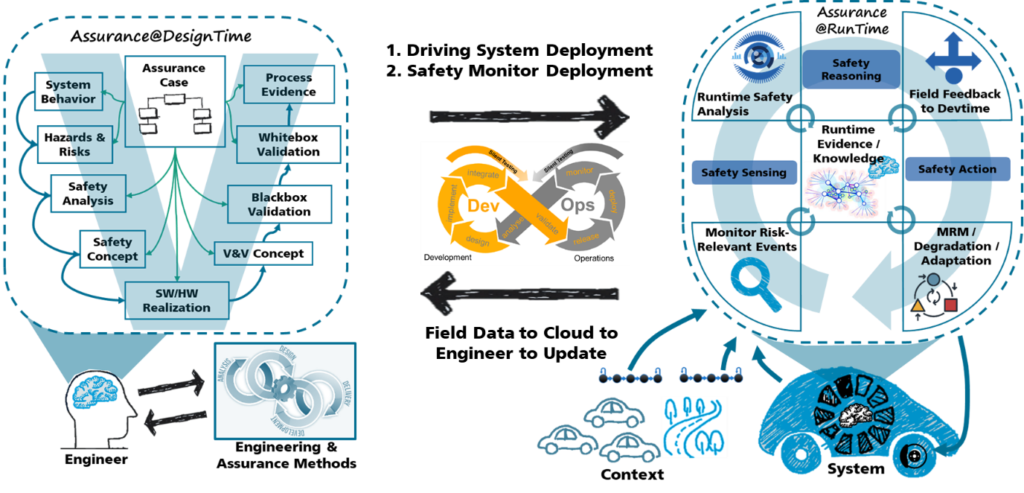
Figure 1 – Dynamic Risk Management Scheme
Such safety monitors come in two flavors, each with a different scope and time scale of reaction. While the first type aims at using the AV fleet to collect and analyze data for building global confidence in the validity of assumptions made during design time safety assurance of the product, the second type may trigger local reactions for single AVs approaching critical situations such as minimum risk maneuvers or graceful degradation. Both monitoring schemes have in common that risk-relevant events need to be perceived during runtime (“Safety Sensing”) and that perceived information needs to be aggregated (“Safety Reasoning”) into a basis for local or global safety action. Apart from monitoring events happening in the environmental situation, the AV system should also be able to dynamically assess its own safety-related capabilities (and, in the future, the capabilities in the context of collaboration scenarios such as platooning) because these might be subject to dynamic change as well. This overall scheme is called “Dynamic Risk Management” [1] and aims at providing dynamic safety assurances.
Note that dynamic assurances are not supposed to replace static assurances that can be generated before deployment, but rather to complement static assurances with evidences that are observable only at runtime. Moreover, dynamic assurances can be used to get rid of worst-case assumptions, which typically impact system performance and availability. Instead of always acting for the worst case, the system is now enabled to perceive the actual situation and reason about it from a safety point of view. In doing so, safety can be ensured while other performance-related system properties can be optimized at the same time.
Whereas all of this comes with an inherent complexity, there is a huge potential to be unlocked in applying dynamic risk management approaches. Future systems will not just be autonomous – they can also be safer, better, and greener. To this end, different properties need to be managed and enforced on different levels. For instance, the safety and performance of a single AV might be optimized on an operational level; the safety and throughput of a complex intersection might be optimized between AVs and the traffic infrastructure; and on the scale of an urban area or even a state, emissions could be managed to be within accepted thresholds. Dynamic assurances, runtime models, V2X communication, and integration of diverse edge and cloud services are among the ingredients needed to make this vision reality.
Dynamic assurance challenge structure
In order to realize such a dynamic risk management system and address the challenges that come with building and assuring it holistically, it is useful to decompose the activities. One way to perform this decomposition is to consider creation time, consumption time, and purpose of evidence as criteria (see Figure 2):

Figure 2 – Classification Structure for Dynamic Assurances
- Design Time Evidence for Design Time Assurance
This activity area is the most familiar one from traditional systems as it addresses the creation of a safety case that systematically lays out hazard identification, safety requirement derivation, capability realization, verification/validation, and safety management, possibly according to the requirements defined in existing standards such as ISO 26262 or ISO 21448. For AV safety cases, such structures need to be extended with AV risk acceptance criteria, more sophisticated context modeling and analysis methods, and the harmonized integration of existing standards with emerging AV engineering and assurance standards and frameworks (e.g., UL 4600, ISO/AWI TS 5083, ISO/TR 4804). German flagship research projects of the PEGASUS family addressing challenges in this regard are “V&V Methods” (https://www.vvm-projekt.de/en/), “SetLevel4To5” ( https://setlevel.de/en/) and “KI-Absicherung” (https://www.ki-absicherung-projekt.de/en/). Most importantly, static assurance methods need to be seamlessly integrated with dynamic assurance methods to establish a fully traceable and continuous safety case.
- Runtime Evidence for Design Time Assurance
Given the number of assumptions that need to be made during design time to make the AV engineering problem tractable, in particular by decomposing the operational design domain into a set of scenarios, it is indispensable to have both technical and organizational capabilities in place to generate empirical evidence in order to gain confidence in the validity of the assumptions made. In addition, unforeseeable environment evolutions such as new traffic actors, new traffic laws, or changed behavior of traffic participants need to be systematically detected and fed into an analysis and reaction tool chain. In order to generate runtime evidence to strengthen design time safety cases, the most important challenges include deciding what to monitor for in the vehicles (i.e., which triggers are deemed “interesting” to be further processed in vehicles with limited storage capacity) and how to build up the infrastructure to trigger change based on the data. This second topic encompasses a lot of open research and practical challenges, as it touches V2X communication, fleet data storage and analysis, and organizational processes for changing and re-certifying safety-critical software quickly after risk-changing triggers, possibly via over-the-air updates.
- Design Time Evidence for Runtime Assurance
If additional functionality is realized in AVs with the sole purpose of monitoring and controlling risks after deployment, it is indispensable to generate design time evidence that the runtime monitors can be trusted, too (bottom left of Figure 2). Here, particular methodological support for analyzing runtime risk and capability variability and developing dynamic safety concepts is required. In addition, V&V strategies need to be developed that can efficiently provide evidence that socio-technical safety concepts (i.e., combining technical systems with organizational processes) work as intended. At Fraunhofer, several approaches have been developed in the last decade to address the problem of seamless integration of dynamic risk and capability monitoring into established design time safety engineering approaches (Conditional Safety Certification and Dynamic Safety Capability Monitoring [2,3], Situation-Aware Dynamic Risk Monitoring [4,5])
- Runtime Evidence for Runtime Assurance
Based on risk and capability variability models, i.e. models that relate dynamic knowledge of runtime situation and system state with risk and safety guarantees, runtime evidences can be produced that enable a local risk control (i.e. perform runtime assurance, Figure 2 bottom right). Such a risk control scheme can proactively optimize safety and utility by dynamically looking at, whether sufficient dynamic AV capabilities exist to address dynamic risks. Research-wise, a major challenge lies in systematically considering both aleatoric and epistemic uncertainty types for controlling risk.
[1] Schneider, Daniel; Trapp, Mario (2018): B-space: dynamic management and assurance of open systems of systems. In J Internet Serv Appl 9 (1). DOI: 10.1186/s13174-018-0084-5.
[2] Schneider, Daniel; Trapp, Mario (2013): Conditional Safety Certification of Open Adaptive Systems. In ACM Trans. Auton. Adapt. Syst. 8 (2), pp. 1–20. DOI: 10.1145/2491465.2491467.
[3] Reich, Jan, et al. “Engineering of Runtime Safety Monitors for Cyber-Physical Systems with Digital Dependability Identities”. International Conference on Computer Safety, Reliability, and Security. Springer, Cham, 2020.
[4] Feth, Patrik (2020): Dynamic Behavior Risk Assessment for Autonomous Systems. Dissertation. Technical University Kaiserslautern, Germany.
[5] Reich, Jan, et al. “Towards a Software Component to Perform Situation-Aware Dynamic Risk Assessment for Autonomous Vehicles”. DREAMS Workshop (Dynamic Risk Management for Autonomous Systems) co-located with European Dependable Computing Conference 2021.